Understanding the Differences between Data Cleaning vs. Data Cleansing in Business Intelligence
In Business Intelligence (BI) field, data is the lifeblood that drives insights, decision-making, and strategic planning. However, raw data is rarely perfect. It often contains errors, inconsistencies, and gaps that can undermine its value and reliability.
This is where the processes of data cleaning and data cleansing come into play. Though the terms are frequently used interchangeably, they entail distinct activities that collectively ensure data quality.
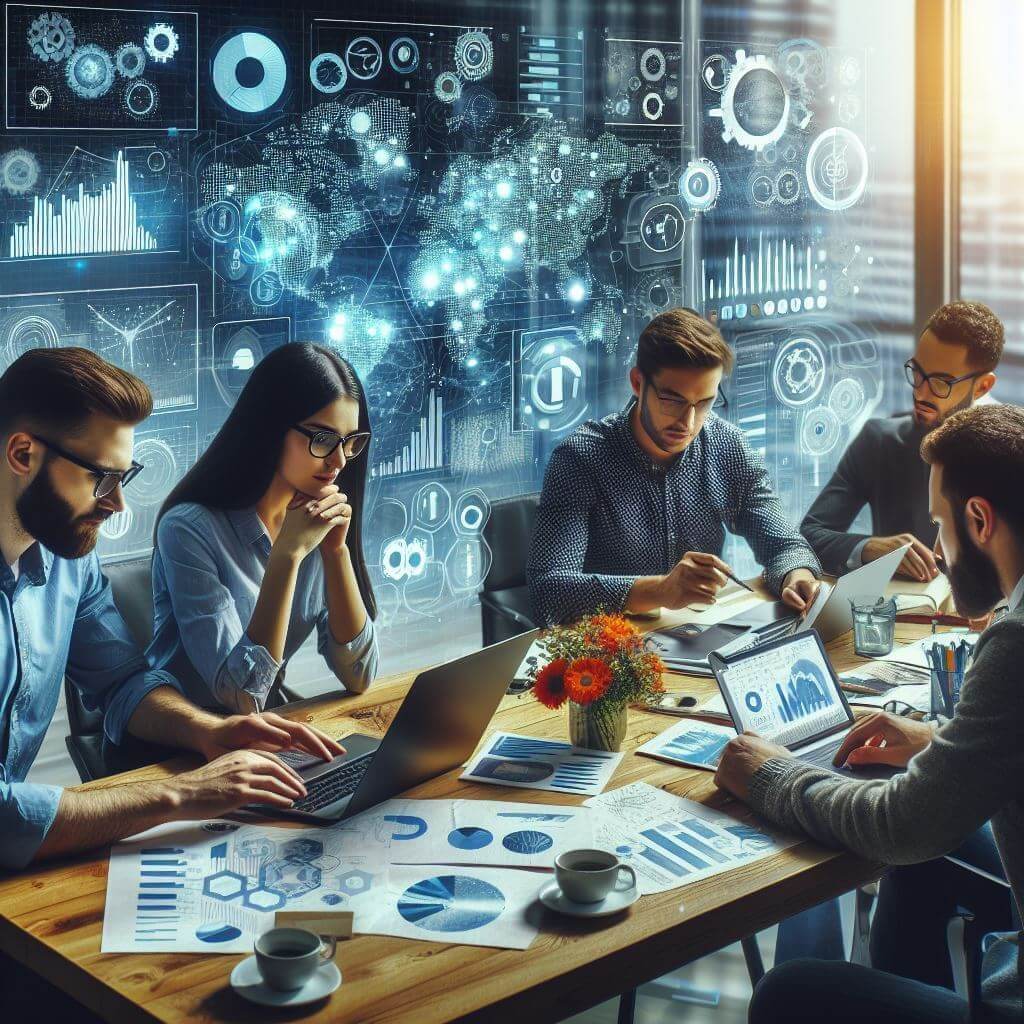
In this article, we will explore the key differences between data cleaning and data cleansing, highlighting their importance in the BI world.
What's Data Cleaning?
Data cleaning is the process of identifying and correcting errors in a dataset.
- It focuses on making the data accurate and consistent by removing or rectifying issues that can compromise its integrity.
- The primary goal of data cleaning is to ensure that the dataset is free of inaccuracies and inconsistencies.
Key Activities in Data Cleaning:
- Removing Duplicates: Identifying and eliminating duplicate records to ensure each entry is unique.
- Correcting Errors: Fixing typographical errors, spelling mistakes, and other inaccuracies.
- Standardizing Data: Ensuring data follows a uniform format, such as standard date formats (MM/DD/YYYY) or consistent capitalization.
- Handling Missing Values: Addressing gaps in data by filling in missing values or removing incomplete records.
Examples:
- Correcting a misspelled customer name.
- Standardizing date formats across the dataset.
- Removing duplicate entries for the same transaction.
Data cleaning is essential because inaccurate or inconsistent data can lead to faulty analyses, misguided business decisions, and lost opportunities. By cleaning data, organizations can enhance the reliability and validity of their BI efforts.
What's Data Cleansing?
Data cleansing goes beyond the basics of data cleaning. It encompasses a broader range of activities aimed at ensuring that data is not only accurate and consistent but also complete, relevant, and properly formatted for analysis. Data cleansing involves more sophisticated methods to validate and enhance data quality.
Key Activities in Data Cleansing:
- Data Cleaning: As outlined above.
- Data Enrichment: Adding missing information from external sources to make the data more comprehensive.
- Validation: Ensuring that the data conforms to defined business rules and standards.
- Normalization: Adjusting values measured on different scales to a common scale, ensuring comparability.
- Transformation: Converting data into a suitable format or structure for analysis.
Examples:
- Enriching customer data by adding demographic information from a third-party source.
- Validating email addresses to ensure they follow the correct format and domain.
- Normalizing financial figures to account for inflation or currency conversion.
Data cleansing is crucial because it not only corrects errors but also enhances the overall quality and usability of data. By cleansing data, organizations can ensure that their analyses are based on comprehensive, accurate, and relevant information, leading to more reliable insights and better decision-making.
The Importance of Data Quality in BI
In today's data-driven world, the quality of data directly impacts the effectiveness of BI initiatives. High-quality data enables organizations to:
- Accurate and comprehensive data provides a solid foundation for strategic planning and decision-making.
- Clean and well-organized data reduces the time and effort required for data analysis.
- Enriched and validated data offers deeper insights into customer behavior and preferences.
- Reliable data leads to better business outcomes and higher returns on BI investments.
Conversely, poor data quality can result in flawed analyses, misguided strategies, and missed opportunities. Therefore, investing in robust data cleaning and cleansing processes is essential for any organization that aims to leverage BI for competitive advantage.
Conclusion
While data cleaning and data cleansing are often used interchangeably, understanding their distinct roles is crucial for maintaining high data quality.
- Data cleaning focuses on correcting errors and inconsistencies
- Data cleansing including cleaning, enrichment, validation, normalization, and transformation.
Both processes are vital for ensuring that data is accurate, consistent, and ready for analysis. By prioritizing data quality through effective cleaning and cleansing, organizations can unlock the full potential of their BI initiatives and drive better business outcomes.